Lazy TIFFs
If you're running into memory-limitations when working with large datasets, you can lazy-load or memory-map the file so that it looks and behaves as if it were loaded, but actually loads data only when needed.
Memory-mapping and lazy loading
Loading lazily is very similar to Reading TIFFs, except with the addition of the mmap=true
or lazyio=true
flag. The differences between the two will be described in the next section.
A good general rule is to preferentially use mmap=true
. It will generally be faster and, perhaps even more importantly, will have much better worst-case behavior. However, it's more limited in the types of TIFFs that are supported, e.g. compressed and/or striped TIFFs are not supported. For those files, use lazyio=true
, which is more flexible.
See the Caveats and important details section for more details.
First, let's look at a demonstration using mri.tif
from the tlnagy/exampletiffs
repo.
using TiffImages
img = TiffImages.load(filepath; lazyio=true);
Regardless of the size of the file, this is likely to return img
almost immediately. The trick is that the data are not actually loaded (yet)– the image data will be loaded on an as-needed basis.
The lazily-loaded img will behave much the same as a normal eagerly-loaded image:
size(img)
(128, 128, 27)
Display the 2nd slice
img[:, :, 2]
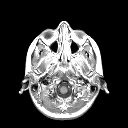
Lazy operations
One of the primary benefits of lazy-loading is avoiding unnecessary work for portions of the image that may never be accessed. I recommend using MappedArrays
to continue the "laziness" of operations.
using Colors
using MappedArrays
eltype(img)
RGB{N0f16}
We can lazily modify our data for only the slices we end up actually displaying. For example, img
is stored as an RGB, despite the fact that you can see it consists only of grayscale intensities. Let's convert the eltype
lazily:
gray_img = of_eltype(Gray, img);
Then when we extract a slice from disk, it converts only that one to gray
slice = gray_img[:, :, 1]
We can check to make sure its eltype
is correct:
eltype(slice)
Gray{N0f16}
Example: Maximum intensity projection
It's pretty straigtforward to do a max-intensity projection:
dropdims(maximum(gray_img, dims=3), dims=3)
While this demonstration was performed with lazyio=true
, for files that support mmap=true
the results would be similar.
Caveats and important details
Mechanism, format support, and performance
mmap=true
and lazyio=true
correspond, internally, to two different strategies for deferring the work of loading, and the differences can be visible to users.
lazyio=true
uses an internal single-slice data buffer, and each frame of the TIFF file is read into this buffer on an as-needed basis.mmap=true
uses memory-mapped I/O to set up a virtual address space for the entire array, and the operating system's memory manager takes care of loading chunks of data into physical memory and mapping it to the virtual address space on an as-needed basis.
The two strategies support different features and exhibit different performance:
mmap=true
requires a one-to-one correspondence between what is on disk and what is in memory. Consequently, features like compression are not supported. Currently, there is also no support for files that write slice data in strips. Both of these features are supported withlazyio=true
.- with
lazyio=true
, switching slices is an expensive operation, because an entire new slice has to be read into the buffer; as a consequence, access patterns that stay "within-slice" (likesum(img[:,:,1])
) are fast, while access patterns that cross slices (likesum(img[1, 1, :])
) are slow; access patterns that alternate between slices (e.g., interpolation across the third dimension) are essentially unusable.mmap=true
can be more selective about the data it loads, for example reading subsets of single slices. It can also keep previously-loaded data in memory even as you switch slice planes, so that reloads are less common. Consequently, when supported,mmap=true
achieves good performance more consistently.
Assigning values and writing to disk
Aside from generality and performance, there are other important differences. Currently, lazyio=true
does not support assigning new values to the array: img[:, :, 2] .= 0.0
throws an error. mmap=true
can support setting values, but setting values also writes those same values to the disk file. To guard against unintended data corruption, by default load(filepath; mmap=true)
opens the file with read-only permission, and then assigning values throws an error. Using flagler.tif
from the tlnagy/exampletiffs
repo (a file which is of a format that can be read with mmap=true
), we get
img = TiffImages.load(filepath_flagler, mmap=true)
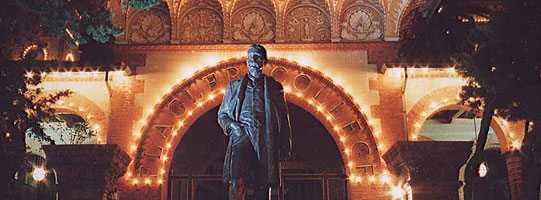
but
julia> img[1,1,1] = RGB(1, 0, 0)
ERROR: ReadOnlyMemoryError()
To support writing, open the file with read/write permissions:
img = TiffImages.load(filepath_flagler, mode="r+", mmap=true);
Then, assignment will work and the same value will be written to the disk file.
Casual use of mode="r+"
can lead to data corruption, so use it only when you intend to rewrite data.
Behavior on Windows
On Microsoft Windows, one additional caveat is that deleting or replacing a file that has been mmap
ed by your Julia process will result in either a IOError: unlink(<file path>): permission denied (EACCES)
or LoadError: SystemError: opening file <file path>: Invalid argument
error. If you're done using the image, you may want to ensure it is garbage-collected first:
img = nothing
GC.gc()
If successful, this will terminate the mmap
and the file can be deleted without causing an error in the Julia session. However, any reference to img
by any other object can prevent garbage collection; one safe pattern is
let img = TiffImages.load(filepath; mmap=true)
# operations on `img` go here
end
GC.gc()
Such tricks are not generally necessary on other platforms, which handle deletion by unlinking the file from its name but otherwise keep the data on disk if it is being mmap
ped by one or more processes. After all such processes have exited, the actual data are deleted by the operating system.
Incremental writing
TiffImages
also supports writing to a file via an append operation. We have a special type for this called LazyBufferedTIFF
, that we can create via the standard empty
function
using ImageCore # reexports Gray and N0f8
img2 = empty(LazyBufferedTIFF, Gray{N0f8}, "test.tif")
32-bit LazyBufferedTIFF{Gray{N0f8}} 0×0×0 (writable)
Current file size on disk: 8 bytes
Addressable space remaining: 4.000 GiB
For data-integrity reasons, TiffImages
will not allow you to append to an pre-existing file and will throw an error if a file exists at the filepath that you provide.
Say you have the following data:
slice = rand(Gray{N0f8}, 256, 256)
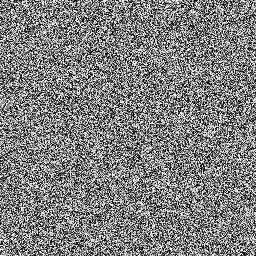
You can then push new data to the img2
object and it will eagerly write that data to disk.
push!(img2, slice)
32-bit LazyBufferedTIFF{Gray{N0f8}} 256×256×1 (writable)
Current file size on disk: 64.152 KiB
Addressable space remaining: 4.000 GiB
The first slice sets the XY dimensions of the TIFF and subsequent slices must have the same dimensions as the first.
push!(img2, rand(Gray{N0f8}, 256, 256))
32-bit LazyBufferedTIFF{Gray{N0f8}} 256×256×2 (writable)
Current file size on disk: 128.297 KiB
Addressable space remaining: 4.000 GiB
The memory-mapped object also behaves like an array and supports most array operations (other than inplace mutating ones like setindex!
)
size(img2)
(256, 256, 2)
To read a slice that you just wrote:
img2[:, :, 2]
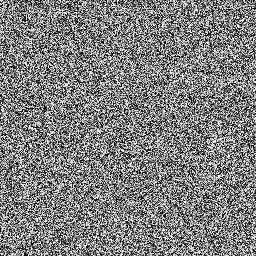
XL files
If you're going to be writing lots of data to disk (4GB+) then it can be helpful to set the bigtiff
flag to true so that TiffImages
can use 64-bit offsets. You'll see that the addressable space sky rockets:
img3 = empty(LazyBufferedTIFF, Gray{N0f16}, "test.btif"; bigtiff=true)
64-bit LazyBufferedTIFF{Gray{N0f16}} 0×0×0 (writable)
Current file size on disk: 16 bytes
Addressable space remaining: 16384.000 PiB
This page was generated using Literate.jl.